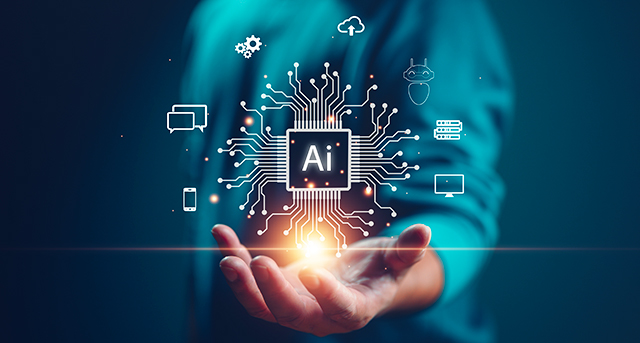
Panelists
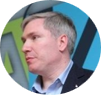
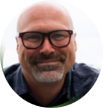
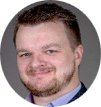
We’ve had a chance to focus on a great deal of speculation and interest in Artificial Intelligence models over the past several shows and for this episode, we asked Dan Geater to join Matthew Heusser and Michael Larsen to help get to the bottom of what AI is actually doing, why the spike in interest, and how testers can prepare and benefit from the AI gold rush currently underway.
Michael Larsen (INTRO):
Hello, and welcome to The Testing Show.
Episode 136.
AI in Testing
This episode was recorded on Thursday, April, 6th 2023.
In this episode, we asked Dan Geater to join us and help us get to the bottom of what AI is actually doing, why the spike in interest, and how testers can prepare and benefit from the AI gold rush currently underway.
And with that, on with the show.
Matthew Heusser (00:00):
Welcome back everybody to another episode of The Testing Show. Now we have had a particular focus on AI technologies, what they’re doing to testing, what’s gonna happen with testing, how to test them, and I think that was a good use of our time and one guest we haven’t been able to have on. It just hasn’t worked out with their schedule (he is based in the UK) and our schedule, we gotta get his perspective… is Dan Geater. Welcome back to the show, Dan.
Dan Geater (00:29):
Thanks for having me back.
Matthew Heusser (00:31):
Longtime friend of the show, I’ve worked with him on consulting projects in the past. He’s been on, I don’t know, half dozen times, something like that. Always appreciate his perspective. And your role has changed. This is a Qualitest full-timer, I’m not sure, became a VP at the company a while back, but very hands-on, practical, doing. Can you tell us a little bit about your role now at Qualitest, Dan?
Dan Geater (00:55):
Yeah, sure. So I’m the VP of Delivery for AI Projects in AMEA for Qualitest. And what that really means is where our customers are getting involved in using artificial intelligence in their quality efforts. Those projects tend to come through a team specialist that sits under me, the data scientists in tests. They’re jointly skilled in data science and also the world of software quality. And those projects can be different kinds of things. They can be customers that are implementing machine learning for themselves and they wanna know, “How do I know it’s good?” One of the big questions that industry still has is, “How do you test AI?” They may be customers that are trying to dip a toe towards machine learning and they’re understanding where do I get started? So implementation wise, they may be customers that are traditional software quality assurance, quality engineering, digital engineering that are trying to optimize or improve their quality, applying machine learning. So things like, “How do I test faster, smarter, better, more effectively? So I spend a lot of time helping understand what are the problems, what can the technology do and how do we help achieve success with this fascinating family of new technologies.
Matthew Heusser (02:06):
Yeah, and that’s really what we’re looking for is someone who has that broader perspective, not just, “I played with ChatGPT once. I know a lot about the testing industry and can guess”, but I’ve worked or have seen, observed, managed a larger number of things that fall under the umbrella of AI so we can extract trends. So I’m super excited.
Dan Geater (02:31):
Happy to help.
(02:31):
Of course we’ve got Michael Larson as usual, our chief bottle washer and co-host, the showrunner. Lots of things.
Michael Larsen (02:39):
That’s a good description. I’ll take it. . Yeah,
Matthew Heusser (02:42):
. We need to work on that. Dan’s like, “This is Michael, right?” For Michael. It’s just started as show producer but really morphed into really anything the show needs and I appreciate it. So…
Michael Larsen (02:51):
Second banana and beginner voice I guess. In fact, if it’s okay, I’d like to ask a fairly generic question because that’s my role here, . And I guess the way that I would start this is to say, without going into too much of an overview of what is AI, I think we’ve covered that significantly in other podcasts. But why should I as a tester, why should I care about what AI is, what AI does, and how should I position myself to understand and learn more? And I realize that could be a podcast all its own .
Dan Geater (03:27):
That is a very nebulous question, there’s
Michael Larsen (03:30):
True.
Dan Geater (03:31):
actually three questions in one. What I’ll say in the first instance, you know, AI and machine learning technology, they’re not new. They’ve been here for a very, very long time, but they’ve really started getting a huge amount of traction in the last couple of years and this is why there’s so much buzz around them. What really seems to have happened is industry’s got the teeth into it. If you look at where AI is right now, where the funding comes from, who’s backing the releases of the big projects, the DALL-Es large language models like GPT, it’s because industry has started to understand what academia has known about for a long time and it’s really started snowballing. And that’s kind of why it’s important right now. Why should you care? It’s the way things are gonna go. Now there are certain problems the AIs we have today are not really suitable to solve.
(04:25):
One of the common questions that we have to answer with our customers is, “I wanna use AI for this. Okay, why are you sure that’s the right thing?” You know, there are still occasions when traditional scripted efforts or traditional approaches work better and there are occasions where AI gives you unique power, particularly the generative AI that can start to tackle new challenges or things like the image recognition AI that let us deal with problems that traditional tools have failed on. So why do you care about it as a tester? There’s two reasons. One is it’s gonna start turning up in more and more of your products. And whilst there are certain things that traditional software testing has learned a long time ago that can and should be applied to machine learning-powered systems, there are also certain things that are different. And so testers need to be aware of those differences to test them effectively.
(05:19):
But the other side of it is there are software challenges that we still struggle to pin down with current tools. So prime example of this will be for example, how do I test unautomateable interfaces? How do I test things like games or maps or medical systems that defy a lot of traditional automation tools? Well machine vision starts trying to unlock this for us. Other challenges might be how do I deal with a very large wordy specification document and understand all the tests I need? Yes, we can read it, we can generate it, but AI can speed us up. AI can optimize us. We can start to look at things like the language models, ChatGPT, the Bards, the so on to understand if they can speed things up for us. They don’t necessarily replace us as testers. That… I’ve yet to see a single AI that can do the job of a tester, but they change the way we think about it the same way automation changed the way we thought about test execution. AIs, as they are today, let us think about different ways of test design, test approaches and test planning and management. I appreciate that was a slightly rambling answer. I hope it gave a little bit of context on why these things are important.
Matthew Heusser (06:31):
That feeds right into our next question, which is how is AI changing the face of testing? So you said it’s changing test design.
Dan Geater (06:41):
Mm-hmm ,
Matthew Heusser (06:42):
which makes sense to me, even with my limited exposure, I can go in and say, Hey gimme a bunch of test ideas. One actual functional use of those tools is, “here’s a table of this thing, here’s a table of those things. Do a transformation and generate me test ideas” where, what’s the intersection of these things? Are you seeing that happen? Does that make the test design effort smaller? What’s the net benefit of that? Which again, I ask you three different questions that are vague ambiguous.
Dan Geater (07:12):
No, it’s ok. So I think I’ll try and do the questions in order. Certain more traditional test problems. Traditional uses of AI in testing are not new. I think the last time I was on the show a while back, we spoke about how testing could already be sped up in terms of prioritization, planning, execution, and where and what do I test using Machine Learning. What’s getting a lot of momentum at the moment is the rise of generative AI and the large language models, the names of these things give away. You know, generative AI is AI that is used to generate things, “given this description, what can you get me?” That wonderful logic that everyone was playing with about three or four months ago where they turned all their avatars into, “Give me my profile picture in the style of a 1980s sci-fi movie poster.” What that’s actually giving you is the ability to take a description, transform it, translate it, and generate it.
(07:58):
And so when you’ve got those things like, “Here’s my table of stuff, here’s my business requirements. Help me determine the testing.” One of the things you do see though with the generative ads and with the large language models, it’s, they’re not flawless. When you actually look at the businesses that are really starting to apply these kind of big machine learning models, these kind of big systems, whilst they give you the ability to, I would say block in and skeleton a lot of what you’re doing, like if you want to go on a one of the language models to generate test cases, you can do this. But I think you do still need the test engineer to come in and make sense of it. Has it really covered the negative case correctly? Has it really covered the edge case correctly? When you look at these systems as they work, what you can see is they can generate an awful lot of standard content for one of a better term.
(08:45):
But when you ask them to think about the more advanced things, so the stuff where you really need to come in and consider, but what if I have this thing and this thing and this thing? How will that apply? So where I see these things working is building out the basics of a lot of what you’re doing. But I think there’ll be a change in what the test engineers do to take those basics, use them as a foundation and then they need to apply kind of the advanced thoughts on top of it to make sure we cover the edges of the corners. An analogy that I like to use for this is I’m the generation of testers that really saw the rise of software test automation. And one of the things that we discovered as we were doing it was test automation made your testing an awful lot faster by definition, but it didn’t necessarily make your testing better.
(09:34):
Even using things like the advanced record playback tools in some sorts, you still need to go in and deal with the framework design yourself. The component, mentalization the componentization of your tests for yourself. And that was where the automation engineers came in as a discipline. They would turn around and say this is what you can and cannot do. Artificial intelligence currently can generate that. And the big models that everybody seems to be talking about is the buzz at the moment, chat GPTs and so on. They will streamline the ability to generate a lot of things. They may prompt testers to think about things they hadn’t considered, but I don’t see them being the be-all and end-all at the end of your testing process. That make sense?
Michael Larsen (10:08):
Yeah, it definitely does. Shifting gears just a little bit here.
Dan Geater (10:12):
Hmm.
Michael Larsen (10:13):
Again, looking at this from a tester perspective, what problems could AI solve for me as a tester?
Dan Geater (10:21):
Mm-hmm
Michael Larsen (10:22):
We understand that testing is gonna be important and that we’re bringing a lot of our traditional tools to this. What would I hope that this would help me with?
Dan Geater (10:33):
The way I tend to think about this is the software testing lifecycle. Much like the software development lifecycle plan, design, test execute and so on. At each stage of that lifecycle there are things that artificial intelligence can help with. Some of them are already done today. We have a number of customers that already use AI to plan the execution orders of their test. The generative AI, things like chatGPT -pilots and the similar models will already be used to start the block and flesh out code that is required in your actual test case designs and implementation. There are other uses of AI around the general test design. So for instance, you can use things like reinforcement learning and genetic algorithms, which are areas that are not new in the world of AI academia, but they’re not really so big in industry to help you generate new test design.
(11:27):
Some of the bigger research organizations have already started doing this, but generally they don’t have anything like retraction at the other big models. There’s a big rise of machine learning and things like looking for correlations, looking for observability patterns to understand what’s happening when the code is out the door and can I use that in my ops monitoring? And this is where the entire field of AI ops comes in. So you know, you know what that Q server is about to fall over, you wanna spin up another one of those or actually I don’t think you should have shipped this build. Let’s roll it out and push the new one. So depending on where you are in that software lifecycle, there are different families of AI that can help you. You have the uses of NLP way at the very beginning where they can help you understand is this requirement good or bad?
(12:10):
Can I even hand this to a developer reliably and know that they will turn this into good code? Can I trust them? Pass it on to the tester. Then have them generate a good test case. With test design, you can use things like the generative AI to block out your tests for you. But there are other kinds of learning that can do similar things. Reinforcement learners where basically you can build a representation of your application and every time the AI is asked with finding a way through it, pat it on the head. “Good AI, you found a new test case. Bad AI, you didn’t find something I can use”, and have it generate test coverage for you. Almost the way an exploratory tester does. With test execution, there are machine learning classification approaches that could be used to prioritize the order of tests. I’m always interested in finding my failures as fast as possible.
(12:55):
We’ve all seen the DRE curve, it’s been tested on 1 0 1 for decades. I wanna know which tests I have to run first. I’ve got 30,000 tests in my regression pack, I can’t run them all every time. So how do I hone in on the ones that I really like to find something based on my knowledge of what’s changed, my knowledge of what my users use, my knowledge of where do I usually find defects? Experienced test managers do this for the product all the time, but there’s only so many of those and if the test packs get bigger even they can’t do it exhaustively, but machine learning can come in and do this. As you moved further through using machine learning to speed up things like triage,, softwares built by more and more people, diverse teams. I’ve got four vendors on my application here.
(13:34):
I’ve got two support teams and there are opposite ends of the time zone shift. They never actually talk to each other. So how do I triage most effectively? Well, we can use machine learning to come through. They actually, you know what, this bug is a config bug in that component. It’s gotta cut those dots all the way out to the right, like I said, where you can use it. Production monitoring, observation, all those wonderful lines of logging that are going through again and again and again. Help me make sense of this. Is this really gonna be a production instance for me or is it just fluff that’s coming out of a fairly inefficient log framework? All this kind of stuff comes together and these are the various ways you could do it, but there’s no one AI that does all of this. These are small tactical AIs of different types. The kind of AI you use to prioritize tests is not the same kind of AI you use to identify redundant test cases and that’s not the same kind of AI you use to generate new test cases. It’s all about understanding what are the different flavors of AI and how can they be applied to different points in the test lifecycle. Again, that was a very long answer but that particular subject is one that I’m very interested in.
Matthew Heusser (14:37):
Yeah, so I was just on the phone with Blake Link who works with me this morning and he was saying that AI is gonna, my word’s not his, but to summarize it, it’s gonna become ubiquitous. It’s it’s gonna be everywhere. It’s gonna take over a lot of things.
Dan Geater (14:53):
Yeah.
Matthew Heusser (14:53):
I think what we talked about for test design, based on what you said, for companies that have a long boring test design cycle where if you miss something important it could be bad. This kind of trained AI might speed up that part of the process by 50%.
Dan Geater (15:15):
I think that depends on what your starting ground is. That’s an interesting question.
Matthew Heusser (15:18):
Of course it’s gonna be range.
Dan Geater (15:19):
So we’ve seen organizations get 30, 40, 50% faster shift-left by using different AI approaches to the way they prioritize and optimize and execute. But I think there’s a couple of things that determine for you your test design approach and how much faster can you get at a test design stage. One of them is gonna be linked to how much testing do you already have? So I’m working with a mature pack and the majority of my tests already exist and I’ve just gotta block in another one for this slightly tweaked functionality. You’re not gonna get so much roi. But if you think of the organizations where maybe they have legacy stack and you don’t necessarily know everything that’s in the application, you know it’s one of those systems that’s been running around for 25, 30 years, how will we figure out what to test against this? I think in those situations you stand to gain more using things like automated exploration of the application then build and generate the test that come back and form the pack. But test generation speed up, it’s hard to tell but I think it will depend rapidly on where you are. So radically on where you are today, we could share it to you for testing to that application.
Matthew Heusser (16:31):
Yeah, I totally understand. It’s gonna depend on where you’re at. I wanted to sort of see where the boundaries are, where your benefit is gonna be between 10 to 40%, zero to 30%. And I think you’ve given us some ideas there.
Dan Geater (16:44):
But it depends. I think you’ll also see different levels of optimization depending on where you are. Cause each of these things takes place in different parts of the life cycle. So what it’ll really come down to is where’s the slowness that you are replacing? So for example, I had a conversation with a customer and we talk to ’em quite a lot about better planning testing and using machine learning to optimize around it and response to that is, “That’s great but I’m gonna run all my tests anyway so I don’t really need to plan them in a different order. I’m just gonna run them.” So okay, so where’s the sticking point? Well the sticking point for me is actually what happens downstream. Maybe it’s environment contention, maybe it’s defect analysis and handling and then it’s about applying the right tool for the job. And so when you think about how do you speed up test case design, if test case design is not your problem, then why use that ai? That comes back by point at the beginning about is this really the right problem to solve? We see that using AI in the testing life cycle helps organizations get sort of 25, 30, 40% more efficient. But it really depends on are you solving the problem that actually affects this organization? Automating everything is fantastic, but if you’re still gonna fall over because you have a two week deployment process, then automating everything just spends a lot of automation engineer time but doesn’t get you any faster time to market. Make sense?
Michael Larsen (18:02):
Yeah, definitely does. So I guess the next question that would interest me at this point is, “Okay, you’ve sold me. I think this seems like something I should definitely prepare for and get ready to use. So that leads me to my next question…
Dan Geater (18:18):
, how do I begin?
Michael Larsen (18:19):
Would I do that? Where do I start? ?
Dan Geater (18:21):
That’s a great question. I think there’s two ways to look at this. There are applications out there that will already tell you they, they have AI infused this, that the other testing. But my preference, and maybe it’s me as a person, my preference is always go the other way and think about the problem and then figure what you’re trying to solve. So most of the AI models, whilst the model themselves might be proprietary, you know the exact flavor of AI that’s in this that the other might belong to the organization. The general theory is all pretty open. If you just do a search for how do LLMs work, for instance, the kind of model that is behind Bard and ChatGPT or if you do a search for how does unsupervised machine learning work, you will find dozens and dozens of articles, introductory courses that tell you kind of the basics.
(19:09):
Machine learning, you don’t need to become a data scientist to leverage a lot of these things. There are open source toolkits that already allow you to start training and building models on your own local laptop and there are courses out there that will take you through it. But it’s important to understand what is the kind of problem you’re solving for. The way I would look to do it would be find one testing problem you wanna solve. Maybe it’s better prioritization tests, maybe it’s blocking some new test cases based on a spec document and you will find people will already have tried it, but you’ll also find that most tools are available to you. And as you start to understand about four or five big families of machine learning and there are different chapters within machine learning, you know that the family of vision AI is not the same as the family of language AI and that’s not the same as the traditional regression AI and so on.
(19:59):
Start to apply it to yourself and work on it and most of the tools you need are already available, open source and free. However, there are commercial offerings that will get you there quicker. Basically we have them within Qualitest. We have an AI machine learning platform. Microsoft, Google, Amazon, they all have machine learning toolkits available at a paid price that will get you there faster, basically. But the learning itself, the understanding that’s already available. The way to get started in my opinion is always pick the problem you wanna solve, try and understand what kind of a problem is this, is this a problem where I can turn it into a classification problem? The thing is either A or B, help me find a model that does this. Is this a problem that is, “I don’t know the relationship between these data points, but I’m sure there is one.” That’s a different kind of problem. Is this a case of, “I’ve got a bunch of stuff but I need to transform it into another bunch of stuff.” Like Matt’s example of data table examples. That’s a different kind of thing. So think about the problem, how do I turn that one back and then start to find the toolkits that make my sense for that? But unfortunately there’s no shortcut to it. Also the information’s there, there’s not really a quick way to do it. You have to learn some of the stuff.
Matthew Heusser (21:07):
So I just thought of this based on something you said earlier. If we’re looking for a commonality.
Dan Geater (21:11):
Hmm.
Matthew Heusser (21:13):
What these models tend to do is take large bits of generally not superly structured data. I mean if it was Superly structured we could put it in Excel and we could look at things like the medium or the median or the max or
Dan Geater (21:29):
mm-hmm
Matthew Heusser (21:29):
the average. Or we could take some randomization and check some things but not superly structured data and then compile it, condense it almost like a Google search where we spit out some answer that allows us to take action. A good test manager could look at all of the commits in git and figure out the module that’s changing the most. And we talked about that on the show. They could look at all the bugs in bug tracker and they could figure out what’s the buggiest and where those bugs come from.
(22:00):
They could look at all these different source days requirements and come up with requirement document F and requirement document G. They’re not gonna play nice together. The intersection of them is gonna be a bug. These tools generally we can throw all these things at the tool and it’ll spit out something that will save us time.
Dan Geater (22:20):
Mm-hmm
Matthew Heusser (22:21):
or can do a combinatorial expansion. We were gonna talk about machine vision where it might spot a dot on a lung that a human being might not or at least save time or tell you where to look. And we were gonna talk about generative adversarial networks that I don’t know much about, but that can simulate hacking from a security perspective based on
Dan Geater (22:42):
mm-hmm
Matthew Heusser (22:43):
how had hacks have gone in the past. Would it be a reasonable inference to consider that One of the big benefits from these is throw data at it, have it do analysis, help you come up with your next step to do. That’s a big value add and if you automate that, you end up with something like the tools that can find buttons on the screens where we don’t really have a UI affordance and they’re literally just going to an XY coordinate but they’re smart enough to do the OCR and find it and click. Really it’s the same thing. It’s looking at a big set of data, compressing it down and suggesting an action.
Dan Geater (23:18):
Yes, basically. One of the strengths is give it the data and ask it to find a pattern. And one of the biggest strengths of machine learning is finding the patterns I don’t know are there. So I’ll give you an example. Understanding test overlaps is a prime example of this. If I’ve got 25,000 tests in my pack, I’m sure that beyond that point, some of them must overlap, some of them will be redundant coverage and 25,000 tests, even if they’re running in a few milliseconds is still an awful lot of testing. And even if they run quickly, I’ve still gotta pull all the results out. I’ve still gotta make sense of that. I’ve still gotta find the real failures versus the false failures and so on. So how do I go through and understand where the inefficiencies are? I mean I can give it to my test lead, but is my test lead really gonna confidently say that test case 14 is the same as test case 24,768 and not be wrong? We can’t guarantee that, but machine learning can.
(24:11):
We can turn machine learning loose on it and we actually do this with customers and we use machine learning to, “find me something I don’t know”, which is that this test, that test, the other test, they’re the same. You can get rid of them or maybe you can merge them, maybe you can join them and so on. You mentioned other kinds of AI there, some of the vision AI, so we use these with customers who are dealing with systems that defy conventional automation. So you think about something like a game or a medical system or a GIS system, selenium and the associated family of processor-based automation tools are very, very powerful. They’re an incredible thing. They’ve seen some great work on them but they still struggle with certain types of information and rich user interfaces, dynamic user interfaces in particular are quite painful. You’ll often find that testing just say, “You know what? I just, the effort to automate around that is unfeasible.
(24:59):
I’m just gonna keep a bunch of guys in my manual test team who are gonna go and do the visual look and feel.” But machine vision opens this up for us. Machine vision has the ability to take a set of images and say I see something in here. And there are pre-trained models that already exist that are capable of recognizing lots of things. For instance, they’ve been pre-trained on open databases, they already know what a bus looks like, they know what a bike looks like, they know what a flower looks like. You can take those, you can build on them and you can use them to help understand, “Well actually what does something on a screen look like? What does this look like?” And then you can expose that and say, “Actually okay, now I’ve basically got eyes for my automation and now I can start to look at different things.
(25:39):
I can start to focus on different things.” All of these systems can either help us solve problems we don’t know we have or we can’t solve in other ways or they can speed things up. But one of the things that I always try to be very mindful of with them is, are you using AI on this problem just because you want to use AI? And it’s okay to say, “You know, I like the thing I wanna play with it,” but that it’s not the same as saying it’s always the most efficient way of doing it. You are right Matt. Sometimes maybe I can just put all this information into Excel and run a very basic function that achieves the same and it takes me 15 minutes, I get an ugly Excel at the end of it, but I don’t have to get my head around a bunch of data science stuff. But then there are other times where actually the only way you can do it is by taking machine learning to it.
Michael Larsen (26:21):
That’s awesome.
Matthew Heusser (26:21):
Thanks Dan. Yeah Michael, I think tools would be next if, if the listener could come home with, if this is the machine learning thing for Python and this is how I can get ChatGPT free and this is how I can… the reader can come home with a half dozen different tools that they can actually use, open source, I think that would be cool.
Dan Geater (26:40):
A lot of the libraries you need are already out there. An awful lot of machine learning is done in things like care ads, TensorFlow, all of these things are freely available. They’re all open source. There are platforms particularly with the cloud providers. You know Amazon has SageMaker, Google has cloud computation, Microsoft has the Azure COG services. So there are paid things that also designed to help you kind of map AI in and out of your applications, particularly if you’re on cloud centric architectures. But the basic models themselves or the basic libraries, an awful lot of them can be pulled from open source projects on GitHub and the like. And if you’re really feeling particularly cruel towards yourself, you can go find the academic papers and you can re-implement this particular sorting algorithm or searching algorithm from scratch. What you tend to find is that those libraries are already built to help you with most of your needs in a certain family of problems.
(27:33):
So for instance, if you look in something like PyTorch, it’ll have a family of modules that are designed for this type of learning problem and that type of learning problem. And other libraries and models exist for some of the bigger problems. When you start to get a bit more complex is when you wanna play with the really big models, the large language models like GPT, Bard, and so on. Some of those you can’t really download for yourself. You have to go to the hosted provider. There are one or two open source models of that kind of scale, but the ones that everyone’s talking about at the moment, which have Google and OpenAI, the latest ones, they’re really only available via commercial approaches. But within the various families of AI, an awful lot of the knowledge is out there. It’s one of the things that I really like about this space. It’s growing all the time and yes there is a lot of backing coming in from the commercial side, from industry, from business that is furthering the field. But an awful lot of very good quality tools are still available, free and open source and people can just get them and use them. Unfortunately, you do tend to need quite a punchy laptop to do it for an awful lot of them, particularly things like the image and the language model, but an awful lot of the information is out there and the tooling itself.
Michael Larsen (28:46):
Fantastic. Thank you so much for that. I do think however we need to be aware of the clock and the time, so this seems like it would probably be a good point for us to put a pin on this. But what we always love to ask is any of our guests for any closing thoughts or what I like to say is, “What’s your elevator pitch on all of this? If you only had one minute to describe all of this to us, how would you go about doing that?” And of course, how can people learn more about you and talk to you if they’re interested?
Dan Geater (29:16):
? I dunno that I can do it in one minute. I think, cuz you guys would probably realize I’ll talk about this stuff for hours. So what I would say, AI and related technologies are fantastic and powerful. They’re very, very exciting, but they’re not a panacea. They don’t exist in quality. We know that automation wasn’t panacea, performance testing wasn’t panacea, cyber is not the panacea, but they do offer a great deal of power to problems that are otherwise unsolvable. We talk about people, process, tech quite a lot. I saw a quote that I liked the other day, which is, you know, “People and process problems aren’t solved by technology, but without technology those problems can be unsolvable”. And I think AI is a prime example of that. It gives you the ability to do process optimizations that you can’t otherwise do, but it is all about knowing where to apply them. You need to understand the limits of what it can do as well as what it’s very, very good at. And then you will go to great success with using it. How to find out more about me? I have a LinkedIn page. I don’t necessarily post on there as often as I should, but I’m always open to hear more. And obviously, you can hear from Qualitest on our website at qualitestgroup.com. Thanks again for having me on the show guys.
Matthew Heusser (30:24):
Hey, thank you Dan. And I think that’s it. So thanks everybody and thank you for listening.
Michael Larsen (30:30):
Thanks for having us.
Dan Geater (30:31):
Thanks guys.
Michael Larsen (OUTRO):
That concludes this episode of The Testing Show. We also want to encourage you, our listeners, to give us a rating and a review on Apple podcasts, Google Podcasts, and we are also available on Spotify. Those ratings and reviews, as well as word of mouth and sharing, help raise the visibility of the show and let more people find us. Also, we want to invite you to come join us on The Testing Show Slack channel, as a way to communicate about the show. Talk to us about what you like and what you’d like to hear, and also to help us shape future shows. Please email us at thetestingshow (at) qualitestgroup (dot) com and we will send you an invite to join group. The Testing Show is produced and edited by Michael Larsen, moderated by Matt Heusser, with frequent contributions from our many featured guests who bring the topics and expertise to make the show happen. Additionally, if you have questions you’d like to see addressed on The Testing Show, or if you would like to be a guest on the podcast, please email us at thetestingshow (at) qualitestgroup (dot) com.