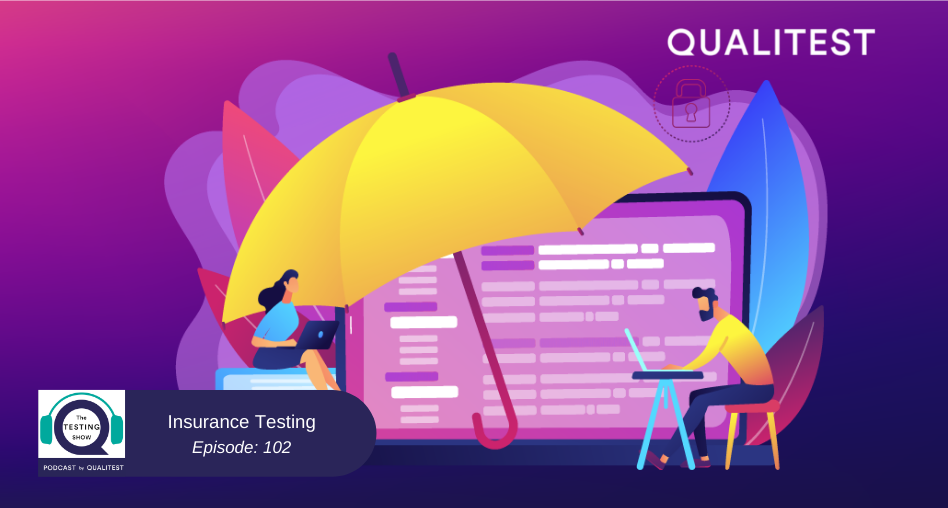
Whether it be auto, home, health, or life insurance, more and more organizations are moving to models where big data, Artificial Intelligence, and Machine Learning are playing a part in determining premiums and claims payouts. There’s a lot of coding happening and a lot of testing opportunities, so listen in and get a bird’s eye view of an interesting area.
Panelists:
References:
- More is More: Big Data is a Big Win for Insurance Companies
- Insurers turn to wearable tech to nudge people towards healthier living
- How The COVID-19 Pandemic Is Fast-Tracking Digital Transformation In Companies
- The Secret Behind Lemonade’s Instant Insurance
Transcript:
Michael Larsen (INTRO):
Hello and welcome to The Testing Show.
Episode 102.
Insurance Testing
In this Episode, Matthew Heusser and Michael Larsen talk with Simon Pickersgill and Anthony White about testing in the world of insurance. As more organizations are moving to models where big data, Artificial Intelligence, and Machine Learning are playing a part in determining premiums and claims payment. There’s a lot of coding happening, devices that share data to help determine premiums, and a lot of testing opportunities.
And with that… on with the show!
Matthew Heusser (00:00):
Thank you, Michael. I always appreciate the introductions. This week, we have Simon Pickersgill and Anthony White, who are at the vice-president level at Qualitest specializing in banking and insurance. So Simon is fascinating in that he actually started his own software testing company (maybe we should do an episode on the business of testing some time) that specialized in banking, insurance, the beginning of the creation of that as a specialized discipline, which was acquired by Qualitest back in 2016. And now he also heads the UK group for e-business. Welcome to the show. Simon, what did I miss?
Simon Pickersgill (00:48):
Hi Matt. Thank you for that welcome. Been in testing and IT longer than probably most of the listeners have been alive. Yeah. Joined Qualitest in 2016 when they acquired the company that I set up in the early 2000s; we were specialists and technical specialists on financial software. There really wasn’t a great deal of discipline around testing. So we were able to bring some techniques and some coding and automation, and it was a very technical discipline back then. More and more tooling might have made the discipline far more user focused over the next decade. Then we’re going back now into a very technical space with what we’re doing. So, yeah, it’s been a joy to be in testing for as long as I have.
Matthew Heusser (01:27):
Okay. We’ve also got Anthony White, who is an AVP at Qualitest in the banking financial services vertical, I guess. Now Anthony worked at Test Direct, which was acquired by Qualitest and before that was in supply chain management. I think the skill set between testing and supply chain management can be very similar. There’s a lot of managing of dependencies involved and planning. Tell us a little bit about that, Anthony.
Anthony White (01:58):
Yes. I started out in logistics and supply chain. So as you said there I was working in the planning department for logistics organization, planning the warehouse, improving, picking, et cetera, managing dependencies. And then I got involved in implementing a warehouse management system for them that then led to organizations where I worked in supply chain. Again, got involved in implementing this time supply chain software. And I think I eventually realized that it’s more testing I was into and the rest, as they say is history.
Matthew Heusser (02:28):
Yeah, you’re not a new convert, but I thought the data point was interesting. Fantastic. And today we’re going to talk about insurance testing. I was really tickled when I heard about this in that it’s not limited to just life insurance, health insurance, or automobile insurance. Usually in my experience, these are kind of treated like their own separate animals, but you found some commonalities between them in the banking industry. Let’s start there if we could, Simon. What makes insurance as an industry unique, especially from a testers point of view?
Simon Pickersgill (03:08):
It’s an interesting question and I am going to defer to Anthony shortly as he’s much closer to the coalface than I am on the actual testing, having just delivered a three-year transformation for a major UK and European insurer, I’m going to say very little in this life is unique. There are particular sets of challenges with insurance, but if we look across sectors, digital transformation and direct to customer trends, use of huge amounts of data and AI tooling. Retail have been doing that for a number of years, certainly in the UK. Now it’s coming into insurance in a big way. What perhaps gives it more of its own flavor is the unique set of regulations and compliance requirements that we need to operate within when we’re assuring the technological change and the use of data in that change. Anthony, if you want to cover a little more detail some of that?
Anthony White (03:59):
Yeah. Thanks Simon. Firstly, the question about what makes it unique. Testing is always transferrable and no matter what sector you’re in, you use the same principles in insurance you would do anywhere else and similar techniques. That said, insurance does have some nuances. Pricing and underwriting is a very specific thing for insurance. Pricing and rating factors, underwriting factors that are applied based on an individual’s circumstances, age, wherever they live, and based on that information pricing or rating factors should be applied or not applied. So it’s important to test those things and ensure you’ve got your boundary value testing in place. Positive, negative testing, and of course it all needs to be automated. And when I first started out, this was all done at the UI level, but very much now it’s moved to the API level. Another thing that’s happening in insurance at the moment shows a moving away from brokers, looking to become more independent. So they’re building their own digital channels integrating with the aggregators. So in the UK, that’s compare the market. There’s methods of getting your brand out there to huge amounts of customers, insure hosted pricing, bringing pricing away from intermediaries and back in-house. And with those digital channels comes website and mobile app testing. So you need to identify ways to complete that testing efficiently and quickly. So again, automation, but you need to be able to complete that on different browser and device combinations, which is a challenge. Technology regularly changing. So you need to keep up with that. Correspondence for insurance is big. So back in the days, letters used to get sent out to customers. These days it’s more emails, SMS, or you can access your documents via portals. Obviously you need to make sure that all the information on there is correct. So checking the static content, the dynamic content that is relevant to an individual such as personal details all needs to be right, and you don’t want to fall foul of any compliance regulations. And then there’s the standard backend policy and claims management. So managing midterm adjustments, cancellations, renewals, handling car payments, individuals want to be able to pay for their insurance over longer time periods. So that’s where your finance calculations come in your APR and those types of things. And that brings us nicely on to third party integration with organizations that handle direct debit, so card payments and working with them to make sure that the quality’s right. I’ve rambled on but I think I’ve I’ve given you a good flavor of different testing challenges at the moment.
Matthew Heusser (06:35):
There’s a lot there. I was at an insurance company long time ago, decade and a half. We did pricing and underwriting. A lot of that was looking at things like standard deviations and histograms and means and medians and there was some science to it, but it was just a lot of math and Excel pulling data out of databases. I would guess if I heard you correctly in retail, we’ve learned to use machine learning to make these sorts of predictions. Then we have to sort of back test the machine learning to see if it got it right. And that’s one of the offerings we’re talking about here.
Simon Pickersgill (07:18):
That’s absolutely correct, Matt. If you take your health insurance experience from the old days and look at it now, there’s so much information available and there is intelligent tooling, machine learning, AI tech, that can analyze that data in the health space now, more and more of the sort of leading providers are moving to wearable technologies and IOT devices. It’s the same in motor, it’s the same in home, but let’s stay with the health analogy. You can have a heart monitor or a Fitbit, whatever means of monitoring polls the device connected to a data source that is monitored by your insurance company. And it will send you messages and advice on behaviors which are going to impact your health and so impact your premiums. So effectively, the insurance company is managing its own risk by advising its people to change their more risky behaviors. They’ll pay less premiums than if they continue with the risky behavior.
Michael Larsen (08:14):
That’s a trip. What I’m curious about… tThe whole COVID 19 situation definitely sent everybody for a loop and opened up all sorts of things that I’d never considered before. In the sense that once we were all kind of grounded and a lot of people were furloughed from jobs or working from home or making the best they can do with it. I discovered a whole world of car theft that I never knew existed. And that is catalytic converters from Toyota Prius’s and other vehicles because my daughter got hit by that. We had no idea what was going on. She went out and started her car and it started screaming. “Uh, what the heck is going on?!” I looked under the car and I realized the catalytic converter was gone. And there’s a whole rash of those going on. Before the pandemic. I’d never even heard of such a thing. Now it’s kind of front page news. So I can only imagine that COVID has incentivized crime or disruption or breakage, fill in the blank, you know, wherever insurance would be needed. So I can only imagine that COVID has forced the insurance industry as a whole to adapt in a way that is very unique. So I was curious if you had any insights on what that impact has been and how the industry as a whole is dealing with it and how we as testers might be able to get an advantage from that?
Simon Pickersgill (09:36):
Yeah, absolutely. Crime as an industry is adapting and surviving because the cars are easy to get to. They’re not moving around. They know where they’re going to be day in, day out, they can watch them and they can take the catalytic converters far more easily from people more regularly than when they’re regularly in use. It’s interesting in insurance as well because they took a real hit from COVID. Deloitte survey, somewhere online that surveys a lot of major insurance company executives. And at least half of them their businesses more in no way prepared for the storm that was to come. They’re in a state at the moment where they’re transforming their businesses to be modern digital insurance companies, but pandemic and casualty losses were huge in 2020. They are expecting something of a rebound this year, but obviously revenues in that space were hit hard and will continue to suffer this year. Life and annuity sales were cut at the pandemic and the interest rate drop. Life Insurance premiums declined by something like 6% in 2020 in the U. S., forecast to recover by about half of that this year. Growth and profitability in annuities and non-life-term products were impacted. And the low interest rate across Europe and in the U S is resulting in significant losses on mortgage loans and et cetera. So they’re having to adapt and survive going into 2021. There’s a need to get ahead of the curve. They’re going to have to continue to spend, but at the moment they have to find that money. So you can see expense management is critical. They’re wanting to do more for less. If I take that more for less down to the sort of testing microcosm that more for less effectively means how can we provide a smarter service that allows them to move at the pace they want to in terms of change, but also within difficult economic times, a service they can still afford. There are ways with the smarter automation that we have as testers, and that Qualitest has a set of frameworks, that sets of capabilities that can speed up testing. And obviously there are ways to balance the locations and job is to bring in far more cost effective testing based on location, but this will allow insurance companies to continue down a change program that if they halt, they will become extinct because they will be obsolete. So they can’t stop, but they need to move forward in perhaps a more cautious way than before because in insurance companies, historically, they’re very wealthy organizations, but this recent pandemic has really hit them hard. Most of the respondents to that survey that I mentioned by Deloitte highlighted the fact that the company’s whole digital and transformation plans were flawed and behind schedule. Not only are they continuing, they’re accelerating their efforts around this. They need to be really clever, smart, and cost-effective. And in knowing insurers at a general level are looking at their talent strategies, not hiring. They’ve now got more of a global market. There’s less of a plan to return to work. I think COVID has proven globally that remote working works. And there was a lot of traditional prejudice in insurance industries against remote working people wanted to look into the eyes and see the people to touch them. That’s gone. Remote working will be here to stay simply because it’s far more cost-effective.
Matthew Heusser (12:49):
Thanks, Simon. Yeah, I think the jury is out on remote work. I’m a little surprised because I am seeing a little bit of a pushback across our customer base of, “We really want you to come into work, please, please, please, please.” And I would expect more of that in the conservative organizations, the banks and the insurance companies.
Simon Pickersgill (13:10):
Not in my experience. We’re talking insurance today, but there’s a massive banking organization we work with that effectively has worked out that how much money they can save by closing offices and have busily closed one of the largest offices in the UK. So big for the work we were doing for them. We set up an office next to it, to service it and provided an overflow. They’ve just closed it during the pandemic and switched to remote working. And we’re seeing insurance companies now looking at less space, smarter hot desking, so they’re not eliminating the office environment and the natural dynamic that comes, but they certainly seem to be embracing that or flexibility to hire wherever and the cost savings you get with it.
Matthew Heusser (13:48):
It would seem to me that there would be an inflection point. And I think we’re on the other side of it, where if 70% of the people are working remote on any given day, there’s not a value add to come into the office anymore. You’re not going to pick up hints by the water cooler, or you’re not going to be on the inside track of a project. There’s not business value add or even so much career value add. You’re not going to rub shoulders with the boss and make the connection. More people are working remote. So that makes sense. One thing we did want to talk on is this use of big data. Insurance companies have had some of the biggest data of the big data because they’ve got claims data and with claims you can make predictions, but Anthony was talking about even more than that. He was talking about personal wearables and lifestyle. So tell me about how that big data is coming up and maybe how we can test it. What special value add can be found in the testing of those predictions?
Simon Pickersgill (14:50):
Big data is a bit an area I’m particularly interested in because it directly links to the AI technology that we were discussing earlier. Data has always been fundamental to insurance companies, but now they’ve got the opportunity to sort of capitalize on the potential of the vast amounts of data they can collect. The ones that are doing this fast enough are getting a competitive advantage in their marketplace. They can use the big data for a number of business critical areas. You mentioned claims there’s underwriting, customer acquisition, customer retention, personalization, pricing, the potential to now analyze oceans and oceans of data at rapid speed allows so much more flexibility. A couple of examples. I think there’s a European insurance agents authority. I forget the acronym for it, but they highlighted recently that policy pricing based on complex risk assessment was one of the biggest uses of big data having the greatest impact. Insurers like IBM and LexisNexis risk solutions and looking to more accurately price each policy holder by individual driving data. You’re not getting an off the shelf policy. You’re getting a personal policy. That product personalization is very powerful in the market and they are able to do this rapidly because they have access not only to all the data they’ve stored, but through open interfaces and access to shared data now across companies. So they can almost instantly using the AI technology price and present a personalized policy. They’ve got these solutions in place. Claims is another area where historically, if you’ve ever made an insurance claim in the past, and I know I have, it can be an awful customer experience because it will take an age. If there’s any complexity to it, it can take weeks to get the money. And occasionally don’t get the money, but big data’s offering insurance providers analytics so they can better segment their claims. And in many cases fully automate them with chatbots, which they’re faster. They’re more accurate on the customer experience. It’s just infinitely better. There’s an example close back to 2016. So it shows how far ahead of the curve. Some companies are it’s American company, Lemonade Insurance. They do general insurance. They do life insurance, one of their chat bots in 2016, settled a claim without any human interaction plugged into the AI engine. In a matter of seconds. That is a very early example of where the industry is looking to go. But it does present challenges in terms of testing, because if it’s settling claims in seconds that shouldn’t be paid, obviously you’re not going to be in business for too long. And so when you’re testing AI and AI models that make decisions around policy pricing around whether to pay claims around fraudulent activity, it’s an AI black box. You don’t have a yes/no right/wrong test. You’ve got analysis based on data, which can very subtle every time it’s run and can evolve over time to be more sophisticated from the machine learning. So the tests that you need, what we call at Qualitest an AI testing strategy. What underpins our AI testing strategy is that we built an AI model. So we have our own AI systems. We have one artificial intelligence system that is configured to then test and provide assurance of AI models for insurance industries in the claims or in the underwriting space. And that allows acceptance and go-live of those sort of products, knowing that no risk involved in going to live. I think everybody’s aware of the early AI systems that were released by Microsoft and Amazon, that when they went live, they had to stop listings because they were reading historical data and coming out with prejudices that was simply the prejudices in the data over time. So you have to assure your data, you have to assure your engine and make sure that your model is doing what is financially and ethically correct for the company. I hope I answered the question.
Michael Larsen (18:51):
Yeah, I think so. Definitely appreciate the insights for that. This is something that has interested me and my forte is social media tools. So that’s kind of what I’ve been working on for the past decade and in the social media space, a lot of things have given way to AI and Machine Learning algorithms. Most things are being run by an algorithm. One of the things that I’ve been recently seeing, and it’s both disheartening and it also makes for a very interesting question and challenge you’ve actually seen on platforms like Facebook and Twitter and Tik Tok. People will go together and mass report an individual because they don’t like them, or they don’t particularly care for what they’re saying. There’s not a human being that’s reviewing going, “Now, wait a minute. What’s going on here?” Now, the AI is going, “Oh, mass reporting, bam!” Shuts the person down and then you have to go back and, “Oh my gosh, what did I, I didn’t do anything!”. And it’s just because of the fact that a prejudice of a handful of people has decided that they can go in and stop somebody from doing something that shows that a concerted group of people can game an AI to work against its intended purpose. And my sneaking suspicion is a concerted effort could also go in to gaming insurance in ways that we might not have considered. I would say that’s kind of a unique challenge that’s currently facing many industries. And I’m curious, how is the insurance industry going to face something like that?
Simon Pickersgill (20:28):
Yeah, absolutely. It is all down to the assurance. So if… I think it was non-deterministic testing, for example. So you built your model. You think your model is okay, everything is configured correctly and looking in and running it. What you need to do before you release it into an environment is effectively have another model that’s capable of coping with those vast amounts of data your model is reading and then highlighting as part of the testing outliers and issues with the model. So you can make your AI model game your insurance model, if you like, to use your terming, you can make your AI model validate the historic data and present trends that may not be palatable for the company that’s putting their AI into insurance. And then you can retrain the insurance AI to validate them out. It’s a learning experience, but you do need to do the assurance first before going to your customer base because, as you say, the models are open to fraud. If you’ve configured your model correctly, there will be AI fraud monitoring your AI claims piece. It’s all done by machines as somebody once said, but yeah, it becomes critical to be confident in the model that you are releasing to your customer base, because if your AI model performs incorrectly or is open to fraud, it will damage your brand and your business. A key part of what we do at Qualitest is brand and business assurance through methods, such as this, not just in the AI space, in all aspects is to ensure that the customer experience remains optimal and secure.
Matthew Heusser (22:02):
So I think we’ve already sort of hinted at this, all the new things that insurance companies are doing right now to changing pricing and underwriting, to make it based on big data, moving away from brokers and moving away from paper documents that come in the mail to electronic correspondence, which is all generated. What are the kind of quality challenges that are going along with that?
Anthony White (22:32):
Okay, so some of the key challenges of insurers at the moment, so they’re making the switch to an introducing digital channels. And I think that’s been sped up by the pandemic and the drive for digital transformation. Organizations are trying to move very quickly and adapt to the customer, improve their CX customer experience and operate more efficiently in this new world. Things like the support tools and chatbots, but they need to evolve and change all the time. So there’s continuous delivery, continuous change in terms of the quality and testing challenges, what we’re seeing our insurers do, that they’re all now moving to Agile ways of working. So they either all have, or they are in the process of converting from traditional waterfall, of course to Agile. Adopting, CRCD implementing thorough automation coverage. There are some of the ways organizations are changing to make sure that they ensure quality. And along with that, there’s some other challenges as well with all of these new technologies, as a test manager in insurance, you’re constantly faced with new technologies. You need to quickly adapt and update your test approaches to make sure you’re thorough testing. There’s browser and device testing solutions in place for that, the test customer experience, you need to consider things like crowd testing to make sure that you can quantify if a change of a release has, improved customer experience or requests. You need to also consider non-functional testing and cyber security.
Simon Pickersgill (24:01):
Oh, sorry, Anthony, on bugs… We were working with an insurance company that is ahead of the curve that has transformed its delivery that is doing continuous testing. The one area that they haven’t included in all this was the cybersecurity that Anthony mentioned. And at the end, they would have, do all the rapid delivery. But before it gets to live, that was a large standalone cyber security phase because the security department and the insurance company, traditional ways of working not keen to open up to testing and to delivery. However that once they found a vulnerability, the whole project would be thrown back. A lot of the benefits of the rapid delivery approach for loss. So we’ve been able to bring cybersecurity into their pipeline of testing with that support. There’s a final checklist at the end for the cyber before it goes live, but the issues and the vulnerabilities and the defects have been found in sprint rather than at a final stage, when they could impact the release to live and released to live If it’s delayed, we can cost businesses millions in terms of new products, particularly if they’re giving up advertising ahead of the go live. And then at the last minute it has to be pulled.
Matthew Heusser (25:11):
The last question we have is what kind of challenges do you think they’re going to face going forward? I guess that’s different than what they currently face. What’s next for the industry beyond the stuff we’re talking about. Do you guys have a good answer for that?
Simon Pickersgill (25:26):
Yeah, that’s a good question. Obviously, the industry is in varying phases of transformation at the moment, depending organization by organization, but they’re all moving pretty much in the same direction. Where’s that direction going to take them post pandemic over the next 10 years? I think they’re going to move to full digital organizations. There’s this new type of economy to underwrite in insurance and more flexible policies. As we talked about personalization, there’s much more dependence on interconnections between distributed systems across the globe, sharing of data, real-time data from the wearable technology. You can see insurers being called upon to revisit that siloed, thinking about insurance products, everything I read and the research and the like points towards almost pay as you live policies that are going to be across the board for motor, for home, for life. They’re all going to be interconnected that you’re going to end up with a motor policy that has an internet box in the car and IOT device in your car. It’ll tell you if you’re going too fast, it’ll impact your motor premiums. If you’re taking routes that the intelligence satnav doesn’t advise you to take, because there’s more traffic, it’s more dangerous. You could see premiums going up on your life insurance, as well as your motor insurance as per the sort of advice from the AI. If you want to live most cost-effectively and perhaps safest-ly and reduce your personal risks, you’re going to have your life dictated for you to a degree in the insurance space by AI and by your monitoring of your behaviors. We talked about the health devices earlier, really in the wearable tech, how insurance companies can also become insurers for wellness, if you like. In the UK, there’s a company, InVitality that do a lot of this already there, out of the curve. We’ve done some work with them. They’ve been very big on the health and fitness piece. It absolutely will continue down that direction so that the fitter you are, the more cautious you are, the cleaner you live, the less you’re going to pay to insurance companies. That can also… Flipping the coin to claims. If you can make claims handle more quickly, better customer experience and making them reliable customer retention driver, I think carriers will adapt new technologies and alternative data sources, but plug them into like the partner ecosystem and adapt their talent models to support sort of technical claim handling with the AI models and the data science. I think Zurich, I know in the UK have teamed up with a car data, so tools that accelerate claims processing and fraud detection so that the skills and insurance companies need are less actuarial, more data science with that go more and more into the sort of non-deterministic testing we talked about earlier, the black box AI testing, where it’s more machines testing machines with the acceptance criteria governed by the human interaction in the testing phase. In general, the future we’ll see insurance sort of shift from where it has been, which is almost a detect, validate, and remediate kind of phase to a predict and prevent, which is everything we talked about with the health devices, the motoring devices, everything is predicting what is a high risk option, and then providing advice to the individual to not do that. And then thus prevent the risk of a claim and the risk to their health. So it’s really going to continue to transform every aspect of the industry. Likewise, to this, and I think Anthony knows more about this than I do the compliance journey that has to come with this.
Anthony White (29:08):
Yeah. So compliance challenges, the facing of anything. So you can categorize specifically related to the pandemic of, is it just generic compliance issues that insurers need to navigate through day to day treating customers fairly? It has been a sudden shift to online sales and claims handling. So operate and new business processes to handle those transactions. And you need to make sure that the customers are being treated fairly across the base. So if you had a business process that was treating customer one way, now that you’ve moved to this digitalized web method, you still treat them fairly. Solvency concerns in this tough climate, insurers need to prove their financial viability. They need to demonstrate that they can survive the pandemic, but also other catastrophic events that might happen as well, flooding or wildfires. For example, if there was to be a big spike to those, can they pay out all the claims on top of living in the COVID world, they’re moving away from COVID in the pandemic. Obviously AI would touch on that before and how AI makes those decisions. So regulators are very keen now to understand what makes AI tick, how is it making decisions? And there’s certain principles that AI technology needs to meet. Simon touched on not discriminating against different ethnic groups or sexes based on historical data and those sorts of things. Then there’s email climate related compliance insurers need to provide affordable insurance. So they need to balance that with the high risk that might be associated with a certain geographic area. And of course, in cyber security, we touched on that before data privacy, it’s important that organizations make sure they have the appropriate cybersecurity testing in place and processes, and that they’re doing everything they can to use the customer’s data responsibly. There was a lot of recent legislation that came in. So GDPR in the UK, IFRA-17 is the new one at the moment in terms of testing, everyone needs to make sure that they’re not utilizing live data in our test systems. It should all be obfuscated.
Matthew Heusser (31:17):
Yeah. Wow. There’s a lot there. So we have insurance for lifestyle, more accurate, better, closer, good deals, aligning of incentives. We have the compliance work, cyber work, and I did not realize that that was going to become law in the UK, the anonymization of test data. That’s going to be challenging. Thanks.
Simon Pickersgill (31:44):
Yeah, it already is. We are moving to a world where machines are going to govern our behavior or we’ll pay for not listening to the machines, but also more and more as we abdicate decisions to machines, we have to increase regulation. And the insurance industry is rapidly moving to bring legislation in. That has to be when we’re assuring these technical systems. We have to show that they are compliant with constantly changing legislation. The more abdication to machines, the more regulation will come to ensure that it doesn’t get out of control. It’s just going to be a mushrooming volume of change, that more and more cleverly we have to test to allow the speed of change that these companies need to be successful. A lot of the sources we’ve quoted and spoken about is on the Qualitest website that will be available in the end of the podcast. Details where to go. Feel free to reach out directly to myself or Anthony. Our details will be on the podcast too. We’re more than happy to explore any of the themes with anyone who is interested.
Michael Larsen (32:48):
All right, Matt, I think that’s close to a wrap. Do you want to do a formal bye?
Matthew Heusser (32:53):
Thanks everybody for coming. Really enjoy it. Look forward to having you back some time. Thank you.
Anthony White (33:00):
Thanks.
Simon Pickersgill (33:01):
Thanks Everybody.
Michael Larsen (33:01):
Thanks for being here.
Michael Larsen (OUTRO):
That concludes this episode of The Testing Show.
We also want to encourage you, our listeners, to give us a rating and a review on Apple podcasts, Google Podcasts, and we are also available on Spotify.
Those ratings and reviews, as well as word of mouth and sharing, help raise the visibility of the show and let more people find us.
Also, we want to invite you to come join us on The Testing Show Slack channel, as a way to communicate about the show.
Talk to us about what you like and what you’d like to hear, and also to help us shape future shows.
Please email us at thetestingshow (at) qualitestgroup (dot) com and we will send you an invite to join group.
The Testing Show is produced and edited by Michael Larsen, moderated by Matt Heusser, with frequent contributions from our many featured guests who bring the topics and expertise to make the show happen.
Additionally, if you have questions you’d like to see addressed on The Testing Show, or if you would like to be a guest on the podcast, please email us at thetestingshow (at) qualitestgroup (dot) com.